Computational sentiment analysis of an online left ventricular assist device support forum: positivity predominates
Introduction
With heart failure rates growing across the world, an increasing number of patients are requiring intervention resulting in a greater number of patients on continuous-flow left ventricular assist devices (CF-LVADs) (1,2). Since the approval of certain CF-LVADs for destination therapy, patients have both been increasing in total number and time on support with a mean survival of 7.1 years on these devices (3,4). With these growing numbers, achieving excellent survival rates is no longer the main goal as survival time increases and patient numbers grow. Clinicians are now targeting means to reduce morbidity and device complications. While clinicians have focused on reduction of complications such as driveline infection, stroke and pump thrombosis, what clinicians believe to be of paramount importance to patients may or may not be at the forefront of patient experience. Further, in a modern age of medical information where patients often search for concerns on the internet prior to seeing physicians, clinicians must be aware of how patients perceive their medical choices and conditions (5). Physicians have the opportunity to utilize online discussion forums in order to understand patient sentiment and how this sentiment might affect patients’ overall health. Evaluation of these online forums may provide new clues into the patient experience, and provide insight into what clinicians may need to address to improve care of not only patients’ physical health but also their mental well-being.
In an effort to seek out shared experiences, patients have turned to internet forums to gain further information about their condition and provide support to each other. This type of behavior has been deemed an effective means of addressing patient-specific concerns for patients going through malignancy (6). These topics, centered on quality of life, may not rise to the need for a clinic visit but remain at the forefront of a patient’s mind. For this reason and more, the creators of MyLVAD.com, a website devoted to LVAD care and patient experience, note their mission is to help improve the quality of life and outcomes for people living in the LVAD world by hoping to provide information, support, direction, and inspiration for those who live with LVADs (7).
As a hub of LVAD patients, caregivers, and physicians, MyLVAD.com, the only forum entirely dedicated to the care of the whole LVAD patient, contains a wealth of information for patients and clinicians alike. Given it is both a means of patient-to-patient communication and a forum for patients and caregivers to discuss their care with physicians, MyLVAD.com represents a unique intersection of care that may present new data on patient psychosocial well-being and overall health. While a patient will likely remember to mention specific clinical symptoms at a doctor’s appointment, the structure and time constraints of such a visit often precludes the patient from speaking about other aspects of life which might be tangentially affected by LVAD support. As this forum is an archive of written patient experience, evaluation of the opinions on MyLVAD.com may provide new clues into the patient experience and guide physicians on how to better approach issues within the LVAD patient population. While the interpretation of the current study’s analysis is not directly proportional to patient experience, it provides a more tangible understanding of the experience than the objective patient outcomes regularly measured in the medical field. We performed a sentiment analysis that pools together discussions from MyLVAD.com to develop a cohesive understanding of the LVAD patient experience (8). While it is important to note that these discussion forums will not reflect the entirety of the patient experience, it is reasonable to assume that the topics most important to LVAD patients and caregivers will be present.
Sentiment analysis techniques have been extensively utilized in several fields, such as finance, politics and business. While there are several validated approaches to analyzing the sentiment of words and phrases, the current study uses the lexicon approach (9). This involves the use of a previously generated library of words that have been assigned a score (+1, –1, 0) based on associated positive, negative or neutral sentiment. These scores are then added together to determine the overall sentiment. While medicine has historically been rooted in very objective measures of health, patient sentiments (fears, opinions, thoughts) might elucidate a hidden segment of patient health and wellbeing which factor into these objective outcomes. Thus, applying sentiment analysis tools to the field of medicine is a relatively novel concept that has yet to be thoroughly investigated. By exploring websites like MyLVAD.com, clinicians can gain better insight into the patient experience and tailor treatment to this subset of patients and their needs, both physically and emotionally.
Methods
Search strategy
The LVAD community support website, MyLVAD.com, was chosen as the source for the data due to its active, engaged, and public patient forum. Text taken from this website included titles of individual posts and subsequent comments left by users which were not filtered.
Selection criteria
Posts from April 24th 2017 to October 1st 2019 were selected for analysis. Posts, comments, and titles that were repeated, or were related to surveys, were included in the data extraction for consistency. A “post” comprised of the main entries by the users. Each page has a collection of posts that are submitted by users with each post entitled by the submitter to give other users a general idea of the content of the post. Other users can click each post to read the submitter’s thoughts and even write up their own thoughts as a “comment”. Each comment is also given a title known as a “subject” to give other users an idea of the comment’s content. In order to minimize text selection bias, there were no restrictions on the type of post, comment, or title that was used for the data extraction. However, it is important to acknowledge the implicit bias associated with evaluating a platform in which members voluntarily seek out, and which requires some degree of technologic proficiency in order to use.
Data extraction and critical appraisal
The data extraction process was automated using a custom Python script (version 3.6). The entirety of the community’s discussion pages, twelve pages in all, from April 24th 2017 to October 1st 2019 was chosen for data collection and extraction. Uniform resource locators (URLs) for these posts totaled 228 links. The website was searched using the “urllib” package which allowed the Python script to open MyLVAD.com and read its contents. Once all the links were collected, the Python script then opened each link and then parsed the HTML for the main posts, comments, and titles. The data was then pasted on a text file which was then pre-processed and analyzed. A summary of these libraries and functions can be seen in Table S1.
Text preprocessing and statistical analysis
The data was tokenized using R software (version 3.6). The “TM” library was used to remove all punctuation marks, numbers, and convert all the letters to lowercase for consistency, while the “SnowballC” library was used to remove all extraneous white-spaces. “Stopwords”, which are the most occurring words in English, such as “a”, “is”, and “the” were removed as they are irrelevant in natural language processing. The “Bing” library, a lexicon of pre-established positive and negative words developed by Bing Liu from the University of Illinois at Chicago, was used to assign each of the words in our data set a numerical sentiment value, either +1 (positive sentiment) or –1 (negative sentiment) (10). The “Bing” library is based on the aspect-based opinion mining model which allows for mining of opinions and the classification of those opinions as positive or negative. After the neutral words (words which do not have a positive or negative connotation) were removed and the remaining words received a +1 or –1 score, additional R software packages were used to create informative visual demonstrations of the data. This included bar plots, wordclouds and comparison wordclouds. A summary of the various packages, libraries, and their functions can be seen in Table S2.
Results
After text preprocessing, 66,821 unique words remained, including 4,623 (6.9%) with positive sentiment and 3,248 (4.8%) with negative sentiment. The net sentiment ratio (number of positive words – number of negative words)/(number of total words) was 2.1%. Words with positive sentiment dominated the 20 most commonly used words (Figure 1). The odds ratio of non-neutral words (number of positive words/number of negative words) was 1.42, indicating a less obvious dominating positive sentiment when considering all of the non-neutral words. Word cloud analysis of positive (green words) and negative (red words) sentiments is shown (Figure 2), indicating a more common mention of “infection” [208] compared to other known LVAD-related complications such as stroke [29], bleeding [30], and thrombosis or clot [32]. In contrast, the most common positive word mentions included “like” [317], “good” [285], “well” [226], “thank” [183], and “recovery” [87].
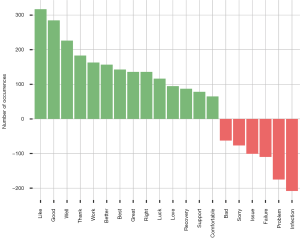
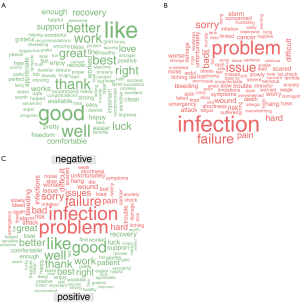
When specifically examining the 20 most common words with either positive or negative sentiment, the following words were mentioned most frequently with sentiment and number of occurrences noted in parentheses: “like” (positive, 317), “good” (positive, 285), “well” (positive, 226), “infection” (negative, 208), “thank” (positive, 183), “problem” (negative, 175), “work” (positive, 163), “better” (positive, 157), “best” (positive, 143), “great” (positive, 136), “right” (positive, 136), “luck” (positive, 116), “failure” (positive, 110), “issue” (negative, 101), “love” (positive, 95), “recovery” (positive, 87), “support” (positive, 78), “sorry” (negative, 77), “comfortable” (positive, 65), and “bad” (negative, 63).
Of these 20 words, a prevailing positive sentiment is demonstrated as 70% of the words are positive. While to a lesser extent, positive sentiment still predominates when considering all words, as evidenced by the positive net sentiment ratio (2.1%) and odds ratio of non-neutral words (1.42). In order to ensure wordclouds remain readable, only the most frequent positive and negative words are included in these figures. To address this limitation, Figure 3 demonstrates the distribution of all non-neutral words analyzed (over 8,000) and represents a color-coated histogram of overall positive and negative word frequencies.
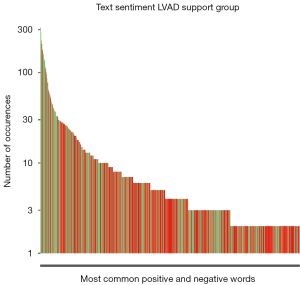
Discussion
With an increasing number of patients being placed on CF-LVADs not only surviving but thriving on these devices, more attention has been focused on the quality of life afforded to this patient population. With this in mind, clinicians find it necessary to focus on patient well-being. These topics include emotional distress following device implantation, cognitive functioning, sleep disruption, sexual activity, driving restrictions, and end-of-life discussions (11). Because these topics are sparsely researched at the current moment, clinicians and patients are left with minimal data with which to discuss these concerns. This leaves patients to turn to websites like MyLVAD.com to have their questions answered. While patients need to be mindful of the anecdotal nature of some posts and responses, ultimately this website is filling a need that patients have. Analysis of the patient and family experience through this website further sheds light on how LVAD patients approach their devices when they leave the hospital. For example, many end-stage heart failure patients suffer from higher rates of depression. Thus, understanding which negative sentiment words are commonplace for LVAD patients may allow clinicians to intervene and mitigate potential negative effects of the device and its impact on the LVAD patient’s health (12). Therefore, careful analysis of sentiment is important to patient care.
In order to counsel patients appropriately, clinicians should be aware of how patients feel globally about their devices. In our analysis, positive sentiment dominated the 20 most commonly used words, which notes that for most patients and their families, LVADs and the experiences related to them are viewed in a positive light. The odds ratio of non-neutral words, defined as the number of positive words divided by the number of negative words was found to be 1.42 (4,623/3,248). This indicates words with positive sentiment were more common than words with negative sentiment. This information is invaluable in the counseling of patients who are questioning whether or not to undergo LVAD implantation. This also provides insight into what patients appreciate about their devices. Positive sentiment could largely be viewed as words related to how patients feel, which could be interpreted as recommendations to other patients, and how the devices have transformed their lives for the better.
Despite the positive sentiment associated with the majority of the most common words from the forum, understanding the negative sentiments related to LVADs is arguably more important to the improvement of the field. The most common negative word in the sentiment analysis was “infection”. Recent studies indicate that infection is one of the leading causes of complication in patients with a rate of 50% and it stands to reason that patients may note this as one of the more common negative sentiments (13). While clinicians are concerned with the reduction of all complications, it appears that patients predominantly discuss infection complications on MyLVAD.com. The mental toll of driveline infections on patients is likely to be great as it often leads to repeat hospitalizations, inpatient antibiotics, wound debridement, and vacuum-assisted closure therapy with device changes two to three times a week both in the hospital and at home (14). These prolonged courses are likely to elicit patient reaction and concern and may reflect why “infection” is prominently discussed among negative words.
Despite being considered among the serious complications to CF-LVAD patients, thrombotic complications, like stroke or pump thrombosis, and gastrointestinal bleeding are not reflected as frequently in patient comments as infection. In order to understand this discrepancy, complications must be viewed in a patient-centered light. In other words, this sentiment analysis reflects how patients are thinking about complications over time. The more frequently a word appears, the more likely it is to be affecting a patient at that time. In a continuous forum, patients are perhaps less likely to frequently discuss complications which happen acutely and are subsequently resolved (as opposed to complications that are on-going). Thus, in certain complications, such as pump thrombosis, a patient will suffer a sentinel event, undergo subsequent treatment (i.e., pump explantation), and the complication will be resolved. As pump thrombosis is acutely treated, the frequency in which it is mentioned over-time is limited. Additionally, complications which require treatments that have minimal impact on everyday life might be mentioned less often as well. For example, gastrointestinal bleeding, while recurrent and rarely resolving without pump explantation, transplantation, or cessation of anticoagulation therapy, can be treated via transfusion, which does not overtly cause undue stress or patient preoccupation when compared to other complications and their treatments. Unlike the daily intrusion of infectious treatment, gastrointestinal bleeding and pump exchange do not represent long-term, repeated, and invasive problems.
However, one complication, unlike pump thrombosis or gastrointestinal bleeding, that can be debilitating and affect patients on a daily level is stroke. A patient who suffered a stroke would likely report an interference to daily living activities much in a similar way to the treatment of a prolonged infection, but it is possible that stroke is less frequently mentioned due to selection bias. A patient with a stroke is less likely to be active on an online forum due to reduction of physical or mental capacity related to this complication.
While clinicians often view infection, gastrointestinal bleeding, pump thrombosis, and stroke as the four major complications of CF-LVAD implantation, it is clear that patients focus on infection as the greatest or most frequent complication. Understanding these categories and knowing how patients express these negative sentiments can inform clinicians regarding which complications are most pressing to patients. Sympathizing with the patient experience is of paramount importance as the field of mechanical circulatory support moves from emphasis on survival to emphasis on survival with quality of life. Shedding light on major patient concerns can guide clinician education efforts, and potentially mitigate patient miseducation through unvalidated online resources.
This analysis should continue to drive engineers and members of the mechanical circulatory support field to search for solutions that mitigate infection in order to improve both patient outcomes and the patient experience. Despite the many complications and obstacles associated with mechanical circulatory support, patients still largely believe LVADs play a positive role in their lives and those of their caregivers.
Limitations and future directions
Given our data is taken from a forum on which patients may choose to post their experiences, there are several biases at play that may affect our data. Predominantly, there is a self-selection bias based on patients who are familiar with using computers or the internet and limited to patients who are functionally able to use these resources. Those who may have suffered a disabling stroke may not be able to perform these tasks, whereas patients who overcame infection, for example, would likely still be able to contribute to the forum. As LVAD patients often develop chronic infection, it represents a persistent burden, as opposed to stroke which represent an acute, potentially debilitating event; thus, the frequency of words may be affected accordingly.
Additionally, posts by patients and caregivers on this website are susceptible to response bias; patients with overwhelmingly positive or negative experiences might be more likely to undergo the extra effort required to create a new post and detail their thoughts. Patients who have experienced complications might be more likely to voice their experience than patients who have not encountered major obstacles to their LVAD support. Alternatively, some patients might experience complications that result in loss of motivation to actively interact with society.
A major limitation of the lexicon approach for sentiment analysis we employed is that each positive or negative word is given an equally impactful score. For example, a particularly negative word, for example ‘death’, and a less severe word, for example ‘nervous’, would both receive a score of –1. Future applications of this type of analysis might be aided by a machine learning approach that can assign a greater distribution of scores based on extent of positive or negative connotation. Another difficulty with this method of analysis is that words are not taken into context but are assigned a positive or negative sentiment independently.
Given words selected from all available titles, body paragraphs, and comments, some data may be taken from posts not directly related to a LVAD-specific issue, falsely elevating the number of positive and negative sentiment words. For example, a forum participant who has an LVAD might write a post concerning their most recent trip to the grocery store in which they list which fruits they like and dislike. Given this is not directly related to the LVAD itself, it falsely elevates the numbers of likes and dislikes obtained from the text. We believe the inclusion of these posts do not profoundly affect our data since these posts are still tangentially related to the LVAD patient experience and likely reflect a general sentiment of life with the device.
In addition to context above, some limitations are reflected in word usage. Words may contain a double connotation that complicate counting them universally as positive or negative. For example, the word “like” may refer to a feeling but may also be used in a simile. Since our analysis conflates both usages into one, it counts both as a positive sentiment. Therefore, our analysis potentially overestimated “like” as a positive sentiment word. Further, misspellings were not accounted for during the preprocessing. Incorrectly spelled words were counted as a separate word, or discarded as a nonsensical word, from their correctly spelled counterparts and may affect our total counts.
Finally, posts that were not written by LVAD patients themselves, such as posts written by their spouses, were included in the data extraction. Thus, the analysis does not specifically contain the thoughts and beliefs of only patients with LVADs, but of also anyone who is close to a patient with an LVAD. We felt that while this minimized the patient-specific nature of our analysis, it provides an all-encompassing patient and family experience with such a device and is still worthy of examining especially given LVAD care often requires both the patient and their caregiver’s intimate involvement.
Conclusions
Evaluation of the patient, caregiver and family experience on the LVAD support group site MyLVAD.com notes that the overall experience denotes a positive sentiment with respect to mechanical circulatory support devices. Infection remains a predominant negative sentiment and concern among patients and their families. Further research is necessary to address the current study’s apparent limitations, notably selection bias, and provide a more detailed assessment of patient and caregiver perspective regarding the multifaceted LVAD experience.
Acknowledgments
Funding: None.
Footnote
Conflicts of Interest: The authors have no conflicts of interest to declare.
Open Access Statement: This is an Open Access article distributed in accordance with the Creative Commons Attribution-NonCommercial-NoDerivs 4.0 International License (CC BY-NC-ND 4.0), which permits the non-commercial replication and distribution of the article with the strict proviso that no changes or edits are made and the original work is properly cited (including links to both the formal publication through the relevant DOI and the license). See: https://creativecommons.org/licenses/by-nc-nd/4.0/.
References
- Cook C, Cole G, Asaria P, et al. The annual global economic burden of heart failure. Int J Cardiol 2014;171:368-76. [Crossref] [PubMed]
- Danielsen R, Thorgeirsson G, Einarsson H, et al. Prevalence of heart failure in the elderly and future projections: the AGES-Reykjavík study. Scand Cardiovasc J 2017;51:183-9. [Crossref] [PubMed]
- Miller LW, Pagani FD, Russell SD, et al. Use of a continuous-flow device in patients awaiting heart transplantation. N Engl J Med 2007;357:885-96. [Crossref] [PubMed]
- Gosev I, Kiernan MS, Eckman P, et al. Long-term survival in patients receiving a continuous-flow left ventricular assist device. Ann Thorac Surg 2018;105:696-701. [Crossref] [PubMed]
- Tan SS, Goonawardene N. Internet health information seeking and the patient-physician relationship: a systematic review. J Med Internet Res 2017;19:e9 [Crossref] [PubMed]
- Cipolletta S, Simonato C, Faccio E. The effectiveness of psychoeducational support groups for women with breast cancer and their caregivers: a mixed methods study. Front Psychol 2019;10:288. [Crossref] [PubMed]
- Boyce S, Christensen D. myLVAD.com. 2019. Available online: https://www.mylvad.com/
- Denecke K, Deng Y. Sentiment analysis in medical settings: New opportunities and challenges. Artif Intell Med 2015;64:17-27. [Crossref] [PubMed]
- D’Andrea A, Ferri F, Grifoni P, et al. Approaches, tools and applications for sentiment analysis implementation. Int J Comput Appl 2015;125:26-33.
- Hu M, Liu B. Mining and summarizing customer reviews. In: Proceedings of the tenth ACM SIGKDD international conference on Knowledge discovery and data mining (KDD’04). New York: ACM Press, 2004:168.
- Maciver J, Ross HJ. Quality of life and left ventricular assist device support. Circulation 2012;126:866-74. [Crossref] [PubMed]
- Celano CM, Villegas AC, Albanese AM, et al. Depression and anxiety in heart failure: a review. Harv Rev Psychiatry 2018;26:175-84. [Crossref] [PubMed]
- Kilic A, Acker MA, Atluri P. Dealing with surgical left ventricular assist device complications. J Thorac Dis 2015;7:2158-64. [PubMed]
- Hernandez GA, Breton JDN, Chaparro SV. Driveline infection in ventricular assist devices and its implication in the present era of destination therapy. Open J Cardiovasc Surg 2017;9:1179065217714216 [Crossref] [PubMed]