Metabolomic profile of patients with left ventricular assist devices: a pilot study
Introduction
Myocardial energy substrate metabolism plays an important role in the regulation of cardiac function because continuous energy generation is required for contraction (1). Metabolic remodeling and bioenergetic insufficiency are common features of heart failure (HF), mainly due to impaired mitochondrial function and energy substrate adaptation in the failing heart (1,2). Previous studies reported impaired mitochondrial biogenesis, proteomic remodeling of mitochondrial oxidative pathways, and altered fatty acids and glucose metabolism in HF patients with idiopathic dilated cardiomyopathy or ischemic heart disease (3-6). Moreover, mitochondrial function has been identified as a potential therapeutic target in HF (7).
To date, data regarding the metabolic profile of chronic HF patients who receive mechanical circulatory support with left ventricular assist devices (LVADs) are scarce. Shahinian et al. reported proteome remodeling associated with post-LVAD cardiac remodeling in patients with ischemic cardiomyopathy (8). Weitzel et al. measured different cardiac metabolites in failing hearts post-LVAD support, and showed that while metabolites related to glucose metabolism recovered their values post-LVAD, amino acid levels, creatine, and the citric acid cycle intermediate succinate, did not show a similar pattern (9). Ahmad et al. showed that greater circulating levels of long-chain acylcarnitines in patients with chronic systolic HF were independently predictive of functional status and mortality, and that abnormalities were modifiable with LVAD support (10). These studies contributed to advancing our understanding of the effect of LVAD therapy upon progression of HF and left ventricular biology; however, they did not correlate changes in the metabolic patterns of LVAD patients with clinical outcomes.
Metabolomics is the systematic study of small-molecule metabolite profiles that specific cellular processes leave behind, and it allows us a powerful new approach to the system biology applied to medicine. The term metabolome is derived from the word genome and refers to the complete set of metabolites in an organism and its organelles, or the total complement of metabolites in a cell (11). In this way, metabolomics refers to the use of analytical methods to identify and quantify all metabolites in a biological system, as well as the monitoring of changes in the metabolome of a biofluid or tissue sample following perturbation (11). In parallel with genomics, transcriptomics and proteomics, the application of metabolomic technologies holds the potential to increase our understanding of pathophysiological processes and consequently the potential to identify novel biomarkers to develop new therapeutic strategies (11). Indeed, the identification and quantification of these low molecular weight molecules (e.g., lipids, amino acids, and sugars) might allow for the definition of the phenotype of a given disease. Accordingly, from a clinical perspective, metabolomics might enhance the understanding of the mechanisms underlying a disease, with potential important implications in terms of personalized medicine (11). Metabolomics began to develop in the 1990s with the pioneering work by Lindon, Nicholson and Holmes (12), and has received inputs from different sciences, including informatics, statistics, analytical sciences, chemistry and system biology. Over the years, it has been applied to several medical disciplines, such as neurology, cardiology, and blood diseases (11-13). Metabolic profiling amplifies changes both in the proteome and the genome, and represents an accurate approximation to the phenotype of an organism in health and disease (13).
With regard to LVAD therapy, the outcome of a patient is often strictly dependent on the ability to adopt a patient-tailored therapeutic treatment. Given this, we hypothesized that metabolomics profiling of LVAD patients could further contribute to identifying novel patient-specific therapeutic targets. To test our hypothesis, in this study we applied untargeted metabolomics to: (I) characterize the metabolic profile of chronic HF before LVAD implantation, (II) evaluate the metabolic signature of the clinical effects of LVAD therapy, and (III) identify potential diagnostic fingerprint biomarkers in the patients’ metabolic profile that may indicate patient-specific susceptibility to adverse events.
Methods
Study design
This is a retrospective study conducted in thirty-three patients with end-stage HF implanted with a continuous-flow LVAD at San Raffaele Scientific Institute in Milan from November 2016 to October 2018. Clinical data were recorded at baseline, i.e., before LVAD implantation, and during follow-up visits. Similarly, to evaluate the metabolic profile, patients had blood samples collected prior to the LVAD implantation, forty-eight hours before the intervention, and post-implant, following at least fifty days of LVAD support. Adverse events were diagnosed according to Interagency Registry for Mechanically Assisted Circulatory Support (INTERMACS) criteria.
Approved by the San Raffaele Scientific Institute Institutional Review Board, the study was performed in accordance with the ethical guidelines of the Declaration of Helsinki; all patients provided written informed consent.
Plasma metabolites measurement
We used the Gas Chromatography coupled to Mass Spectrometry analytical technique (GC-MS) to identify and quantify metabolites in platelet-free plasma samples (PFP). Data mining was performed by Multivariate Chemometrics methods. Models from discriminant analysis were calculated and differences in metabolites between pre- vs. post-intervention were evaluated.
The analytical method has been described elsewhere (14). Briefly, 400 µL of PFP were treated with 1,200 µL of cold methanol in 2 mL Eppendorf tubes, vortex mixed, and centrifuged for ten minutes (min) at 14,000 rpm (16,900 G); 400 µL of the upper phase were transferred in glass vials (1.5 mL) and evaporated to dryness overnight; 50 µL of a 0.24 M (20 mg/mL) solution of methoxylamine hydrochloride in pyridine was added to each vial, samples were vortex mixed, and left to react for seventeen hours at room temperature in the dark. Then, 50 µL of N-Methyl-N-trimethylsilyltrifluoroacetamide (MSTFA) were added and left to react for one hour at room temperature. Samples were then diluted with hexane (100 µL) with tetracosane (0.01 mg/mL) as the internal standard, just before GC-MS analysis. Analyses were performed on an Agilent 5977B GC/MS interfaced to the GC 7890B (Agilent Technologies, Palo Alto, CA, USA), equipped with a DB-5ms column (Agilent J&W Scientific, Folsom, CA, USA). Injector temperature was 230 °C, detector temperature 280 °C, helium carrier gas flow rate was set at one mL/min. GC oven temperature program was the following: 90 °C initial temperature, one minute hold time, increasing 10 °C/min to a final temperature of 270 °C, 7 minutes hold time. Samples (1 µL) were injected in split mode (1:4). After a solvent delay of 3 minutes, mass spectra were acquired in full scan mode using 2.28 scans/s with a mass range of 50–700 Amu. Each acquired chromatogram was analyzed by means of the free software AMDIS (Automated Mass spectral Deconvolution and Identification System;
Statistical analysis
The AMDIS data matrix including 108 metabolites was processed with the SIMCA12plus software. Missing values were replaced with half of the minimum positive values in the original data, and after normalization by sum, data were log transformed and categorized using Pareto scaling for the purposes of analysis, including univariate analysis, partial least square discriminant analysis (PLS-DA), orthogonal PLS-DA (OPLS-DA) (16), and its associated Variable Importance in Projection (VIP) score. PLS-DA models were tested with the leave-one-out cross validation (LOOCV) method for the evaluation of statistical parameters (correlation coefficient R2, cross validation coefficient Q2), which allowed us to determine the optimal number of components for the model description.
Results
Patients’ demographics and pre-operative clinical characteristics are reported in Table 1. Median age at implant was sixty-eight years (IQR: 65–72 years); 31 (94%) patients were male; etiology of HF was ischemic in seventeen patients (51%); seven patients (21%) were on INTERMACS profile one-two, according to INTERMACS classification with TCS-modifier (17); all patients were on NYHA functional class III-IV; intention to treat was destination therapy in thirty patients (91%); ten patients (30%) had diabetes mellitus; thirty patients (91%) were stabilized with short-term mechanical circulatory support prior to LVAD implantation (intra-aortic balloon pump, Impella® or extra-corporeal membrane oxygenation). Fifteen patients (45%) were implanted with the HeartWare HVAD (Medtronic Inc., USA), while eighteen (55%) had the HeartMate3 (Abbott Laboratories, USA). Median time of follow-up at the time of the post-implant analysis was 187 days (IQR: 63–334 days).
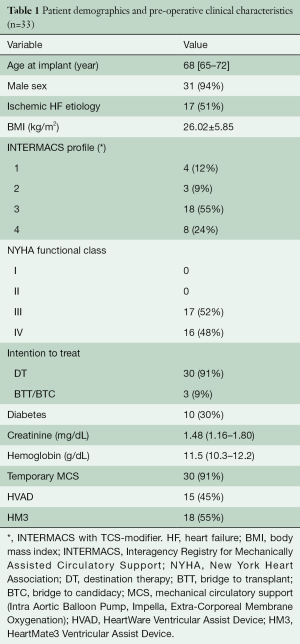
Full table
Figure 1 shows the results of the PLS-DA model. PLS-DA allowed identifying the differences in the metabolomic profile between the pre- vs. post-implant samples; Table 2 summarizes metabolomic differences and changes between the pre- and post-implant samples. According to our results, different important variables (VIP) separating the pre- vs. post-implant samples were identified, belonging to different chemical classes, including: (I) amino acids (alanine, proline and their carboxylation adducts, 4-hydroxyproline, cysteine, threonine, ornithine, glutamine), (II) sugars and reduced sugars (mannose, fructose, glucose, xylitol, sorbitol), (III) carboxylic acids (2-hydroxybutyric, 2-ketobutyric, aminomalonic, gluconic γ-lactone, quinic), and (IV) a purinic derivative. In particular, consistent with the severity of HF, our results show pre-implant reduced amino acid metabolism and increased galactose metabolism, which reversed post-implant (Table 2).
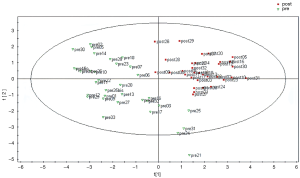
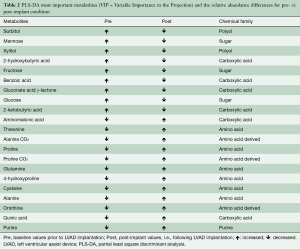
Full table
Data measured post-implant provide a metabolic signature of the clinical effects of LVAD therapy. These changes are associated with improvement of post-implant patients’ functional status; in detail, we observed a significant shift of patients from NYHA class III-IV to class I-II following LVAD implant (NYHA class I-II: pre-implant =0% of the patients; post-implant =97% of the patients; P<0.001).
Moreover, the comparison of the pre-implant metabolomic profile of patients who developed a thrombotic event (stroke; n=4, 12% of the patients) vs. a matching control population revealed a metabolomic fingerprint in this group mainly associated with alteration of fatty acid biosynthesis and mitochondrial beta-oxidation of short-chain saturated fatty acids (Figure 2), and related to altered profiles of octanoic acid, kynurenine, xylitol, inositol, glycine, pseudouridine, 3,4,5-trihydroxypentanoic acid, aminomalonic acid and gluconic acid γ-lactone.
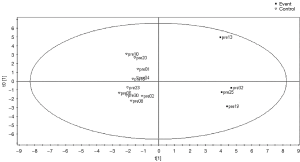
Discussion
We investigated the diagnostic value of metabolomics in the setting of durable mechanical circulatory support in a real-world population of patients implanted with a continuous-flow LVAD. Our results show that, beyond producing the starting point of the metabolic profile of chronic HF patients requiring LVAD implantation, metabolomics has allowed characterization of significant changes in this profile over the course of support. In this regard, this study provides, for the first time, preliminary evidence that LVAD therapy triggers significant changes in the metabolomics of HF concomitant to the clinical improvement of functional status (i.e., NYHA class). Moreover, with this study we describe specific metabolic changes driven by an intervention rather than different baseline disorders or disease severity. While it is reasonable to assume that these changes are mainly associated with hemodynamic recovery offered by durable LVAD support, we also speculate that prolonged exposure of patients’ blood to the artificial titanium surface of the LVAD might further impact metabolomics with respect to the baseline pre-operative profile. Similarly, we speculate that prolonged exposure of blood cells to supraphysiological shear stress might also contribute to metabolomic changes over time. In turn, this might expose susceptible patients to higher risk of developing adverse events; this is consistent with previous studies by our group, where we suggested that shear stress triggers and promotes thromboembolic complications in patients with a baseline prothrombotic profile (18-23).
Furthermore, our results suggest that metabolomics have the potential to effectively identify pre-operative diagnostic biomarkers associated with patient-specific susceptibility to develop adverse events, consistent with the recognition of a metabolomic fingerprint in patients who developed a thromboembolic complication (stroke). This peculiar feature of metabolomics might have important implications as far as (I) the identification of new criteria for patients’ risk stratification, and (II) the definition of optimized, patient-tailored post-operative therapeutic strategies are concerned. Of note, our analysis simply required the collection of peripheral blood samples, which is a fast and relatively safe procedure, and supports effective translation of metabolomics in routine clinical practice.
Our results are in accordance with and further expand upon previous studies, which showed that metabolomic analysis has discriminative value in the prognosis and diagnosis of HF (24,25). It is worth noting that the sampling of blood was performed during routine follow-up, before any adverse event occurred, thus reinforcing the prognostic value of our assumption; indeed, our analysis revealed early signals of equilibrium disruption triggering adverse events. Importantly, the baseline “thrombotic fingerprint” eliminates any possible inference of post-implant therapeutic approach, including (I) pharmacological therapy, (II) differences in hemodynamic characteristics of the patients (MAP, aortic insufficiency, right ventricular dysfunction, etc.) and (III) different hemodynamic characteristics of the LVAD support itself (rpm, flow). Our results warrant further studies aimed at exploring the potential of metabolomics to identify further fingerprints prognostic of different scenarios of LVAD adverse events.
Study limitations
This is a single-center retrospective analysis limited by relatively low numbers of patients; in particular, the prognostic capability of metabolomics to stratify patients at risk of developing thromboembolic complications—as well as other scenarios of adverse events—needs to be validated in larger cohort studies. On the other hand, the single-center nature of this study excludes possible biases associated with inhomogeneous post-operative therapeutic approaches. Moreover, we did not analyze differences in the baseline metabolic profile of patients with different etiologies of HF (ischemic vs. non-ischemic). Furthermore, evaluation of the impact of temporary mechanical circulatory support on metabolomic changes warrants further studies. Indeed, we cannot exclude that temporary mechanical circulatory support may have affected the pre-operative metabolic profile. We also acknowledge that post-implant data were collected over a variable duration of follow-up. Finally, the occurrence of specific metabolic changes right before the clinical manifestation of the adverse event was not investigated, and requires future analysis, as it might significantly improve the prognostic power of the method.
Conclusions
This study investigated changes in the metabolomic profile of chronic HF patients implanted with a continuous-flow LVAD. Post-implant, the metabolic profile was altered as compared to baseline, making the metabolic signature a marker of the clinical effects of LVAD therapy. Furthermore, metabolomic profiling allowed for the identification of diagnostic fingerprint biomarkers indicative of patient-specific susceptibility to adverse events, namely thromboembolic complications. Our findings suggest that the analysis of the metabolic profile can complement currently available clinical tools in managing patients implanted with LVADs.
Acknowledgments
Funding: This work was supported by Fondazione Cariplo (Milano, Italy) grant No. 2015-1044 (awarded to FC).
Footnote
Conflicts of Interest: The authors have no conflicts of interest to declare.
Open Access Statement: This is an Open Access article distributed in accordance with the Creative Commons Attribution-NonCommercial-NoDerivs 4.0 International License (CC BY-NC-ND 4.0), which permits the non-commercial replication and distribution of the article with the strict proviso that no changes or edits are made and the original work is properly cited (including links to both the formal publication through the relevant DOI and the license). See: https://creativecommons.org/licenses/by-nc-nd/4.0/.
References
- Stanley WC, Recchia FA, Lopaschuk GD. Myocardial substrate metabolism in the normal and failing heart. Physiol Rev 2005;85:1093-129. [Crossref] [PubMed]
- Doenst T, Nguyen TD, Abel ED. Cardiac metabolism in heart failure: implications beyond ATP production. Circ Res 2013;113:709-24. [Crossref] [PubMed]
- Karamanlidis G, Nascimben L, Couper GS, et al. Defective DNA replication impairs mitochondrial biogenesis in human failing hearts. Circ Res 2010;106:1541-8. [Crossref] [PubMed]
- Bugger H, Schwarzer M, Chen D, et al. Proteomic remodelling of mitochondrial oxidative pathways in pressure overload-induced heart failure. Cardiovasc Res 2010;85:376-84. [Crossref] [PubMed]
- Dávila-Román VG, Vedala G, Herrero P, et al. Altered myocardial fatty acid and glucose metabolism in idiopathic dilated cardiomyopathy. J Am Coll Cardiol 2002;40:271-7. [Crossref] [PubMed]
- Sack MN, Rader TA, Park S, et al. Fatty acid oxidation enzyme gene expression is downregulated in the failing heart. Circulation 1996;94:2837-42. [Crossref] [PubMed]
- Brown DA, Perry JB, Allen ME, et al. Expert consensus document: Mitochondrial function as a therapeutic target in heart failure. Nat Rev Cardiol 2017;14:238-50. [Crossref] [PubMed]
- Shahinian JH, Rog-Zielinska EA, Schlimpert M, et al. Impact of left ventricular assist device therapy on the cardiac proteome and metabolome composition in ischemic cardiomyopathy. Artif Organs 2020;44:257-67. [Crossref] [PubMed]
- Weitzel LB, Ambardekar AV, Brieke A, et al. Left ventricular assist device effects on metabolic substrates in the failing heart. PLoS One 2013;8:e60292 [Crossref] [PubMed]
- Ahmad T, Kelly JP, McGarrah RW, et al. Prognostic Implications of Long-Chain Acylcarnitines in Heart Failure and Reversibility With Mechanical Circulatory Support. J Am Coll Cardiol 2016;67:291-9. [Crossref] [PubMed]
- Barderas MG, Laborde CM, Posada M, et al. Metabolomic profiling for identification of novel potential biomarkers in cardiovascular diseases. J Biomed Biotechnol 2011;2011:790132 [Crossref] [PubMed]
- Lindon JC, Nicholson JK, Holmes E. The Handbook of Metabonomics and Metabolomics 2007. Amsterdam: Elsevier.
- Mercuro G, Bassareo PP, Deidda M, et al. Metabolomics: A New Era in Cardiology? J Cardiovasc Med (Hagerstown) 2011;12:800-5. [Crossref] [PubMed]
- Barberini L, Noto A, Fattuoni C, et al. The Metabolomic Profile of Lymphoma Subtypes: A Pilot Study. Molecules 2019;24:2367. [Crossref] [PubMed]
- Halket JM, Przyborowska A, Stein SE, et al. Deconvolution gas chromatography/mass spectrometry of urinary organic acids--potential for pattern recognition and automated identification of metabolic disorders. Rapid Commun Mass Spectrom 1999;13:279-84. [Crossref] [PubMed]
- Wehrens R. Chemometrics with R. Multivariate Data Analysis in the Natural Sciences and Life Sciences. Springer Heidelberg Dordrecht London New York, 2011. ISBN 978-3-642-17840-5.
- Stevenson LW, Pagani FD, Young JB, et al. INTERMACS profiles of advanced heart failure: the current picture. J Heart Lung Transplant 2009;28:535-41. [Crossref] [PubMed]
- Valerio L, Consolo F, Bluestein D, et al. Shear-mediated platelet activation in patients implanted with continuous flow LVADs: A preliminary study utilizing the platelet activity state (PAS) assay. Annu Int Conf IEEE Eng Med Biol Soc 2015;2015:1255-8. [Crossref] [PubMed]
- Consolo F, Dimasi A, Rasponi M, et al. Microfluidic approaches for the assessment of blood cell trauma: a focus on thrombotic risk in mechanical circulatory support devices. Int J Artif Organs 2016;39:184-93. [Crossref] [PubMed]
- Consolo F, Sferrazza G, Motolone G, et al. Platelet activation is a preoperative risk factor for the development of thromboembolic complications in patients with continuous-flow left ventricular assist device. Eur J Heart Fail 2018;20:792-800. [Crossref] [PubMed]
- Consolo F, Sferrazza G, Motolone G, et al. Shear-Mediated Platelet Activation Enhances Thrombotic Complications in Patients With LVADs and Is Reversed After Heart Transplantation. ASAIO J 2019;65:e33-e35. [Crossref] [PubMed]
- Selmi M, Chiu WC, Chivukula VK, et al. Blood damage in Left Ventricular Assist Devices: Pump thrombosis or system thrombosis? Int J Artif Organs 2019;42:113-24. [Crossref] [PubMed]
- Consolo F, Pozzi L, Pieri M, et al. Influence of Different Antithrombotic Regimens on Platelet-Mediated Thrombin Generation in Patients with Left Ventricular Assist Devices. ASAIO J 2020;66:415-22. [Crossref] [PubMed]
- Bayes-Genis A, Liu PP, Lanfear DE, et al. Omics phenotyping in heart failure: the next frontier. Eur Heart J 2020;ehaa270.
- Chen L, Song J, Hu S. Metabolic remodeling of substrate utilization during heart failure progression. Heart Fail Rev 2019;24:143-54. [Crossref] [PubMed]